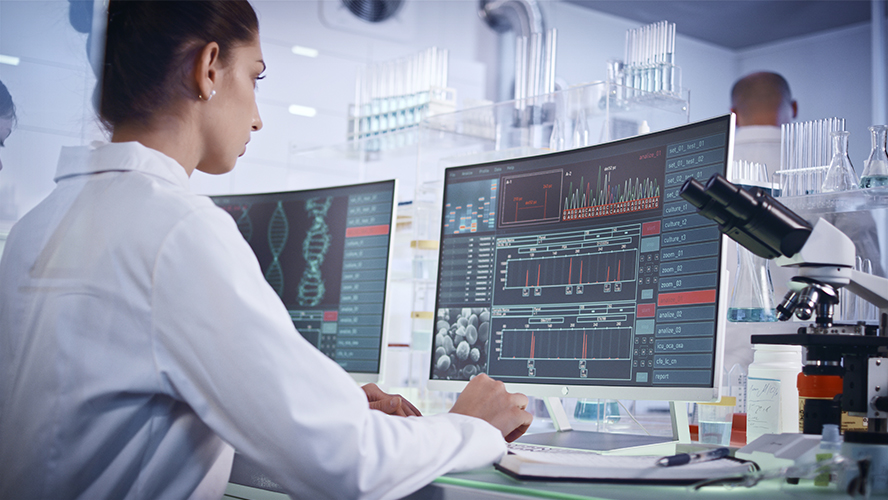
Osteoarthritis (OA) affects a staggering 500 million people around the world. This painful and debilitating degenerative disease occurs when cartilage in the joints wears away, with the knee being the most commonly affected joint. When standard treatments stop working, the only remaining option for those with knee OA (KOA) is knee replacement surgery (called a total knee arthroplasty, or TKA). Sadly, up to one-third of patients experience no relief from this surgery provides no relief.
In a new study from UHN’s Schroeder Arthritis Institute (Schroeder), a team led by Dr. Mohit Kapoor, Senior Scientist and co-senior author of this study, has developed the first method of successfully classifying KOA patients using multiple ‘omics domains (also known as multi-omics) to explain differences in post-TKA outcomes. Using multi-omics and artificial intelligence (AI) models, researchers were able to analyze to multiple sets (domains) of biological molecules simultaneously and investigate connections between them that could not have been in previous research, which looked only at individual domains.
The Schroeder team’s breakthrough classification method (also called a model) is composed of two novel algorithms—one using an AI-based deep-learning technique to identify subtypes of KOA patients (called endotypes) and one using machine learning (ML) to analyze differences between patients within each endotype. "Our model uncovered three novel KOA endotypes and identified key features that likely contribute to differences in patients’ responses to TKA,” remarks Dr. Divya Sharma, senior biostatistician and co-first author of this study. The study further revealed that the factors contributing to whether a patient experiences pain post-TKA are unique to each KOA endotype—as are the molecular pathways responsible for the development of this disease in the first place.
“Beyond deepening our understanding of what underlies KOA and differences in patients’ responses,” says Dr. Jason Rockel, Staff Scientist at Schroeder and co-first author of this study, “we are excited by the possibility of models like ours helping to transform how treatment decisions are made”. Predictive models could be a tool for health care providers to determine whether a particular treatment will benefit a patient before they decide to offer it. Dr. Rajiv Gandhi, Clinician Scientist and co-senior author on this study, adds, “such a tool would streamline the process of finding an effective treatment for an OA patient by eliminating the need for trial and error, ultimately preventing patients from undergoing invasive treatments with long recovery processes, like TKA, without the promise of success”.
By making their data and model publicly available, the team hopes that the greater OA research community will contribute to an even deeper understanding of this debilitating disease. While this is only the first iteration, with further refinement, a tool like the model in this study has the potential to be used in clinics and clinical trials for the KOA community and beyond.
The co-first authors on this study include:
- Dr. Jason Rockel – Staff Scientist at Schroeder Arthritis Institute.
- Dr. Divya Sharma – Senior Biostatistician at Princess Margaret Cancer Centre and Assistant Professor at York University
- Dr. Osvaldo Espin-Garcia – Biostatistician at Schroeder Arthritis Institute
- Katrina Hueniken – Biostatistician at Schroeder Arthritis Institute
- Dr. Amit Sandhu – Postdoctoral Fellow at Schroeder Arthritis Institute
- Dr. Chiara Pastrello – Scientific Associate at Schroeder Arthritis Institute
The co-senior authors on this study are include:
- Dr. Mohit Kapoor – Senior Scientist at Schroeder Arthritis Institute, Professor in the Department of Surgery and Department of Laboratory Medicine and Pathobiology at the University of Toronto, and Canada Research Chair in Mechanisms of Joint Degeneration
- Dr. Rajiv Gandhi – Clinician Scientist at Schroeder Arthritis Institute and Associate Professor in the Department of Laboratory Medicine and Pathobiology at the University of Toronto
- Dr. Yoga Raja Rampersaud – Clinician Investigator at Schroeder Arthritis Institute and Professor in the Department of Surgery at the University of Toronto
- Dr. Anthony Perruccio – Senior Scientist at Schroeder Arthritis Institute and Associate Professor at the Institute of Health Policy, Management and Evaluation at the University of Toronto
- Dr. Igor Juriscia – Senior Scientist at Schroeder Arthritis Institute and Professor in the Department of Computer Science and Department of Medical Biophysics at the University of Toronto.
This work was supported by the Canada Research Chairs Program, Tony and Shari Fell Platinum Chair in Arthritis Research, Campaign to Cure Arthritis, and UHN Foundation.
Rockel JS*, Sharma D*, Espin-Garcia O*, Hueniken K*, Sandhu A*, Pastrello C*, Sundararajan K, Potla P, Fine N, Lively SS, Perry K, Mahomed NN, Syed K, Jurisica I#, Perruccio AV#, Rampersaud YR#, Gandhi R#, Kapoor M#. Deep learning-based clustering for endotyping and post-arthroplasty response classification using knee osteoarthritis multi-omic data. Ann Rheum Dis. 2025 Feb. doi: 10.1016/j.ard.2025.01.012.
*Contributed equally as first authors.
#Contributed equally as senior authors.