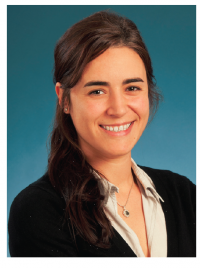
Heart failure (HF) is an exponentially growing medical and economic problem. One in five people over the age of 40 will develop HF in their lifespan. Mortality in patients with HF is higher than in most cancer patients. Currently, the direct and indirect costs of treating HF are approximately 3 billion dollars in Canada. Optimal management of patients with HF is crucial to improve outcomes and reduce costs and depends on adequate assessment of each patient’s mortality risk to decide on appropriate testing and intervention. There are many predictive models, with accurate performance, that can estimate mortality in HF patients but they are seldomly used. The reasons for this limited use are mainly related to the absence of guidelines recommending their use and consequent physician unawareness, the impracticality of a model or difficulty accessing the model or the uncertainty of the model's impact on clinical management. Current practice for prognosis assessment in HF patients relies on physician intuition which has been proven to be limited. The relative performance of clinical intuition and prediction models has not been well studied, but initial work highlights possible limitations of intuition. The extent to which differences in prediction result in differences in patient management and outcomes is even less well studied. Accurate estimation of mortality risk in patients with HF is key to guide management and to allow patients and physicians to make informed decisions about the appropriateness of medical plan.
Some of my current research projects include the following:
- To identify the best available predictive model in patients with heart failure. One of the products of my initial steps as a researcher was the development of an evidence based predictive score, the Heart Failure Meta-Score (www.hfmetascore.org). We have conducted a study to test the Heart Failure Meta-Score’s performance in comparison to the Seattle Heart Failure Model, the most widely validated model, and the SHOCKED predictors, a recently developed promising model.
- To incorporate other predictors such as biomarkers (e.g. N-terminal pro brain natriuretic peptide or troponins) and exercise performance to existing models and evaluate their impact on prognosis assessment
- To compare physician intuition and model prediction to estimate prognosis in ambulatory heart failure patients and to evaluate the relationship between utilization of resources and physician estimates of prognosis
- To evaluate the impact of incorporating predictive models into clinical practice on patient outcomes
- To evaluate outcomes in patients undergoing heart transplantation or mechanical circulatory support