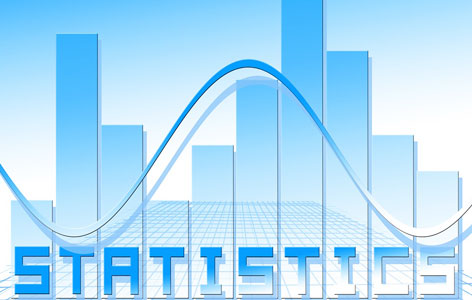
By Krithika Muthukumaran, ORT Times Writer and UHN Trainee
Scientists are in the midst of a reproducibility crisis. One of the factors leading to poor reproducibility is the application of weak statistical tests, including the misuse of the p-value, a common test used to judge if the results are important and the hypothesis is true. Prompted by a growing concern among statisticians on how conclusions are being drawn from research data, this crisis led to the American Statistical Association to release—for the first time since its founding 177 years ago—a set of principles and guidelines on how to appropriately apply the p-value.
The p-value is used to test if the null hypothesis that postulates absence of an effect or difference between two groups is true. It measures how compatible the data is with the null hypothesis, and a lower p-value suggests that your data has evidence to reject the null hypothesis. A p-value equal to or less than 0.05 is generally accepted by the scientific community as being statistically significant. The recent guidelines developed by the Association suggest that this need not be always true: they advise not to make scientific conclusions or policy decisions based solely on p-values. They caution that this is important to prevent muddled thinking, in which p-values are given greater importance than the larger question. There must be full transparency and the data analysis along with the statistical tests used, calculations and data must be reported along with the research finding. The limitations of p-value should be understood and more sophisticated tools, such as Bayesian tests, must be used when feasible.
Proper training and continuously updating your knowledge to improve statistical literacy will greatly help prevent misinterpretations. Nature Methods publishes a monthly column called "Points of Significance" (https://www.nature.com/collections/qghhqm/pointsofsignificance) to aid biologists with basic statistical concepts, statistical methods and experimental design.
Good statistics is part of good science. It means that there is a well thought out experimental design, critical evaluation of possible sampling errors, logical understanding and interpretation of data, and complete reporting. Be aware of both the applications and limitations of statistical tests, adopt the appropriate statistical methods and have more stringent thresholds before claiming success. This would reduce the chances of false positive results and help make sound conclusions.