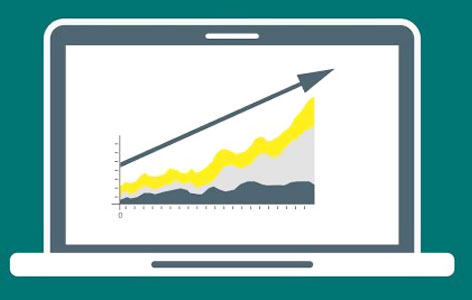
By: Shabana Amanda Ali, ORT Times Writer
It’s Friday afternoon at 4:00pm and you’re sitting in front of your computer with your favourite statistics program ready to go. You’ve just completed the third replicate of a week-long experiment, and the data are all organized for analysis. The results from the first two weeks of experiments were promising, showing the expected trend, but you’re still unsure whether this finding is ‘real’. You enter the data and hope with great anticipation that your P value will be less than 0.05, but it turns out to be 0.06. And your weekend is ruined.
How should you interpret a P value of 0.06? Should you repeat your experiment for a fourth week to increase power and try to show that there is a statistically significant difference? Or does it support the null hypothesis that there is no real difference between your study groups? Either there is or there isn’t a difference between the groups. The hypothesis must be accepted or rejected—right? Perhaps not.
The scientific community has come to rely on statistical significance as the determining factor of the overall significance of research findings; however, a recent Comment in Nature suggests that statistical significance should be retired due to its common misinterpretation. The authors are not advocating for a ban on P values, confidence intervals or other statistical measures. Instead, they recommend improving the application of these measures.
The P value is widely used and reported in the scientific literature as being greater than or less than 0.05, corresponding to a statistically non-significant or significant finding, respectively. While the value of 0.05 is arbitrarily chosen, this value is collectively accepted across the scientific community. This value means that we accept a 1 in 20 (5%) chance that the difference observed between groups is occurring by chance alone. Whether this accept/reject dichotomy is the most meaningful way to interpret research findings is the argument being made by Drs. Amrhein, Greenland, and McShane, the authors of the Comment in Nature.
The authors suggest that P values be reported as the actual value with sensible precision (for example, P = 0.022 or P = 0.14), and that confidence intervals be renamed as ‘compatibility intervals’ where all values inside the interval are carefully interpreted. They explain, “Factors such as background evidence, study design, data quality and understanding of underlying mechanisms are often more important than statistical measures such as P values or intervals.”
Ultimately, this is a call to action for scientists to critically evaluate and explain their research findings rather than rely on a potentially flawed system of statistics to determine the importance of results. After all, observations that do not reach statistical significance may still have biological significance and clinical relevance. Removing the pressure of reaching a contrived statistical threshold, while still enforcing reasonable requirements for demonstrating an effect, would create the space for more creative—and more innovative—interpretation of research findings.